I Am But A Prediction
One clear California winterâs day, a few years ago, I ingested a small amount (honestly, a tiny amount) of psychedelic mushrooms. An hour later, I stood gazing at a familiar five-story building. Its window sills had started morphing. Some grew smaller, others bigger. It was as if the building had come to life. At that moment, my brain was trying to infer the external causes of the pattern of light striking my eyes. But the psychedelic had somehow messed with the whole process. The three-pound mass of tissue inside my bony skull was unable to get a fix on the geometry of the windows and was skewing my perceptions from moment to moment, generating illusory movement where none existed. These shifting shapes were revealing an important aspect of how our brains work.
Crucially, the mushrooms also blurred my perception of myself. I seemed nebulous, far less sharply defined than usual, in a pleasant sort of way. Understanding how we perceive ourselves has long been an obsession of mine. I spent years researching and writing a book on the self, examining the ways in which neuropsychological conditions such as schizophrenia perturb that sense of self, and whether such disturbances provide clues to how we are constructed in the first place. One insight stood out: many aspects of oneâs selfâwhether tied to awareness of our physical bodies, or of being a person with a particular identityâare simply perceptions created by the brain and body. The Scottish philosopher David Hume argued that we are a bundle of such perceptions that are formed with âinconceivable rapidity.â These perceptions cohere to create a complex self, and we see ourselves as such. But how does our brain create these perceptions, and are these perceptions born of objective realities, verifiable to others?
One way to explore this question is to tackle the simpler and more familiar question of visual perception: how does our brain help us perceive our environment, using the light entering our eyes? An intriguing avenue for understanding vision is via the use of optical illusions. Our susceptibility to aberrant perceptions reveals a lot about the brain mechanisms that create them. Another approach is to build artificial intelligence models of human vision and subject them to optical illusions. Are they susceptible too, and if so, what does that tell us about how perception shapes the human self?
Perception as prediction
When it comes to making sense of vision, a theory of perception called predictive processing is gaining ground. Neuroscientists are increasingly of the view that the brain uses prior knowledge about the world to hypothesize (or predict, in the parlance of the field) the external causes of sensory inputs. These could be, for example, predictions about the causes of light patterns or sound waves impinging on our eyes or ears. At each moment, what we perceive is the brainâs prediction and not whatâs actually out there.
Mostly, the theory goes, the brain guesses correctly and our perception is veridical. If it gets it wrong, the brain has mechanisms to correct itself. But sometimes it canât and we perceive things incorrectly, making us prone to illusions and sometimes even psychedelic hallucinations. If predictive processing is the way the brain works, then the morphing window sills, for instance, were caused by erroneous predictions succeeding each other with Humean rapidity, creating the illusion of movement.
Prior knowledge, or priors, about the world can be anything from the things we learn over our lifetimes to knowledge thatâs either learned over evolutionary time or very early in development. While the former may be malleable, the latter can be pretty unshakeable. Such firm priors usually represent knowledge about the physical world and the physics of things in it (our brains are doing some pretty sophisticatedâif intuitiveâphysics, unbeknownst to us). For example, our brains have learned that light comes from above, a nod to the overwhelming influence of sunlight (other forms of light that humans have experiencedâfrom fires to, say, bedside lampsâare comparably recent phenomena). Itâs almost impossible to consciously override such strongly wired knowledge. We also have prior beliefs that buildings donât breathe or sway in the wind like trees, and hence donât see window sills changing shape. That is, until we take an innocently small amount of psilocybin.
Psychedelics aside, there are simple, run-of-the-mill phenomena that we can all experience, which give us insights into the brainâs workings. For decades, psychologists and neuroscientists have used optical illusions to probe the brain. Illusions help us uncover the priors that are built into our sense-making machinery.
Consider these footprints in the sand: the two panels are identical; one is simply an upside-down version of the other.
In the left panel, we see the footprint as an indentation and on the right as having certain protrusions. Our brains take the darkened regions to be shadows and combine them with our expectation that light comes from above, to create that perception. In the right panel, our brains hypothesizeâwronglyâthat parts of the footprint just above the darkened portions are raised, and you can do little about it. This prediction turns into perception.
The modern history of this idea goes back to German physicist and physician Hermann von Helmholtz, who suggested back in the 1860s that our brains must be making such predictions. Helmholtz used his theory to explain a phenomenon called bi-stable perception. Take, for example, something called the Rubin vase (named after the Danish psychologist Edgar Rubin, who devised it). Itâs a two-tone image, consisting of dark silhouettes of two faces looking at each other, with a light-colored gap between them. The gap looks like a vase. But youâll either see the faces or a vase, never both simultaneously.
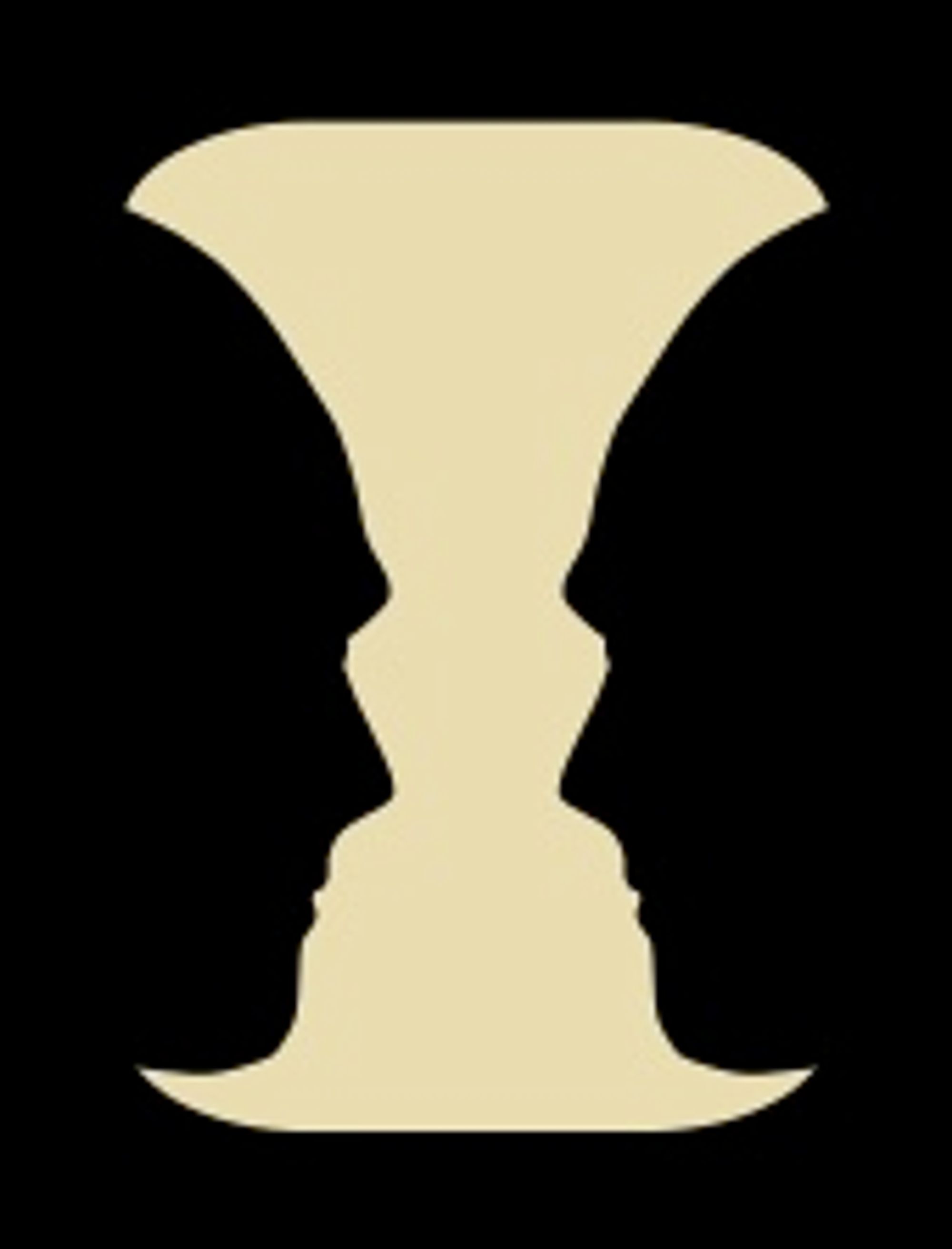
Helmholtz posited that bi-stable perception is best understood as the brainâs effort to explain the causes of all this sensory data. Since both causes are equally likely, our perceptual mechanism keeps flipping between a vase on the one hand and the two faces on the other. Had one interpretation been overwhelmingly more likely, our brain would have settled on it as a stable percept, as it does in the case of the protruding footprints. This explanation goes against the grain of our intuitive sense of perception, and indeed accounts for several crucial neuroscientific impasses.
Not just bottom-up
Until a few decades ago, neuroscientists and even computer scientists who tried to endow machines with vision thought that perception was a bottom-up process. In the context of human vision, itâs instructive to consider one particular pathway in the brain. This pathway, responsible for recognizing objects, begins in the retina and proceeds, brain layer by brain layer, toward the highest reaches of the cortex.
A naĂŻve notion of perception would suggest that each layer of this pathway processes the incoming information, first identifying low-level features such as edges, colors, textures, and shapes, and then putting it all together to form the final perceptâsay, a vaseâin the topmost layers of the visual cortex. Computational neuroscientists have a name for this: feedforward processing. The information flows from the bottom layers of the visual hierarchy to the top, assembled piecemeal at every stage, until it appears in its wholesome fullness in the topmost layer as perception.
This approach to perception is likely wrong. A group of computer scientists discovered this the hard way when they tried to create bottom-up computer vision. The process was computationally intensive. Imagine writing a piece of software to recognize, say, a coffee mug. A brilliant software engineer writes the code that takes input from a camera and recognizes in the image the various parts of the mug, including its handle, and construes that the object is a mug. Then someone comes along and turns the mug around, causing its handle to be hidden from view. Whatâs the machine to do? Thereâs no way for the software engineer to envision every eventuality: every possible position of the mug, every possible lighting condition, and so on. Bottom-up, or outside-in, computer vision doesnât work.
Thereâs another reason to doubt simple feedforward processing. Information in the brain doesnât just flow one way, from the senses to the higher cortical regions. The brain is replete with connections that go the other way, as well. In computational terms, if feedforward connections are those that go from the lower layers of the brain (meaning those closest to the senses) to the higher layers (farther away from the sense organs), then the connections in the reverse direction are called feedback. Neuroscientists have long wondered about these feedback connections in the brain. What do they do? They have no clear, accepted theory to explain it all. There are, however, lots of hypotheses.
Turning perception inside-out
One hypothesis is a formalization of Helmholtzâs thinking. Itâs called âpredictive processing,â a phrase we encountered earlier, and it refers to the general theory that the brain uses prior information to hypothesize the causes of sensory inputs. Neuroscientists tend to use another phrase, predictive coding, to talk about how inside-out predictive processing might be implemented in our brains.
The basic idea is fairly straightforward. The brain is organized into layers, such that the lowest layer is in close contact with the senses and the highest layer is the one that contains the information or representations that we actually perceive, consciously or otherwiseâas the best explanation for whatâs going on lower down in the hierarchy. The intermediate layers are only talking to the layers immediately above and below them. Predictive coding argues that each layer in the brain is making predictions about the activityâor outputs of the neuronsâin the layer below. The use of the word prediction, though commonplace in the field, is somewhat misleading in this context. Itâs not necessarily a prediction about the future. Think of it as hypothesizing about or anticipating the outputs of the neurons in the layer below.
Predictions flow from the top to the bottom, layer by layer. The bottommost layer is being told, in the case of vision, what the outputs of its neurons should be, given that the topmost layer is expecting to see something consistent with prior knowledge. The lowest layer compares the neuronal outputs induced by the incoming sense information to the prediction, and if thereâs an error between the two, it informs the layer above. That layer then corrects the outputs of its neurons to accommodate the error signal, but this likely results in another error signal that has to be percolated upward. And so, the error signals move from the bottom to the top of the hierarchy, again layer by layer.
Whether or not the topmost layer corrects its hypothesis depends on the firmnessâor precisionâof the prior belief: the more malleable the belief, the more likely the error information influences the hypothesis.
While cognitive psychologists slowly came around to accepting Helmholtzâs idea, strong support for it came from computational neuroscientists. In 1999, Rajesh Rao and Dana Ballard developed a simple computational model of predictive coding, in which predictions flowed down (feedback) and errors flowed up (feedforward). It was an explicit mathematical model, equations and all. The model was simple, given the computing power of the late 1990s. Itâd be a decade and a half before it was given its computational due, thanks to a revolution in a field called machine learning.
Machine learning is a form of artificial intelligence (AI) in which machines learn about patterns in data to make inferences about new data. The most dominant form of machine learning today is a technology called deep neural networks. These networks are composed of layers of artificial neurons, where each neuron is an extremely simplified computational model of a biological neuron. It receives inputs, does some computation, and produces an output. In a deep neural network, thereâs an input layer of neurons that receives what could be called sensory informationâsay, the values of all the pixels of an image. The network has an output layer that might signal, for example, â0â to denote the image is of a dog or â1â for a cat. Sandwiched between the input and output layers are multiple other layers, which arenât directly exposed to the inputs or outputs.
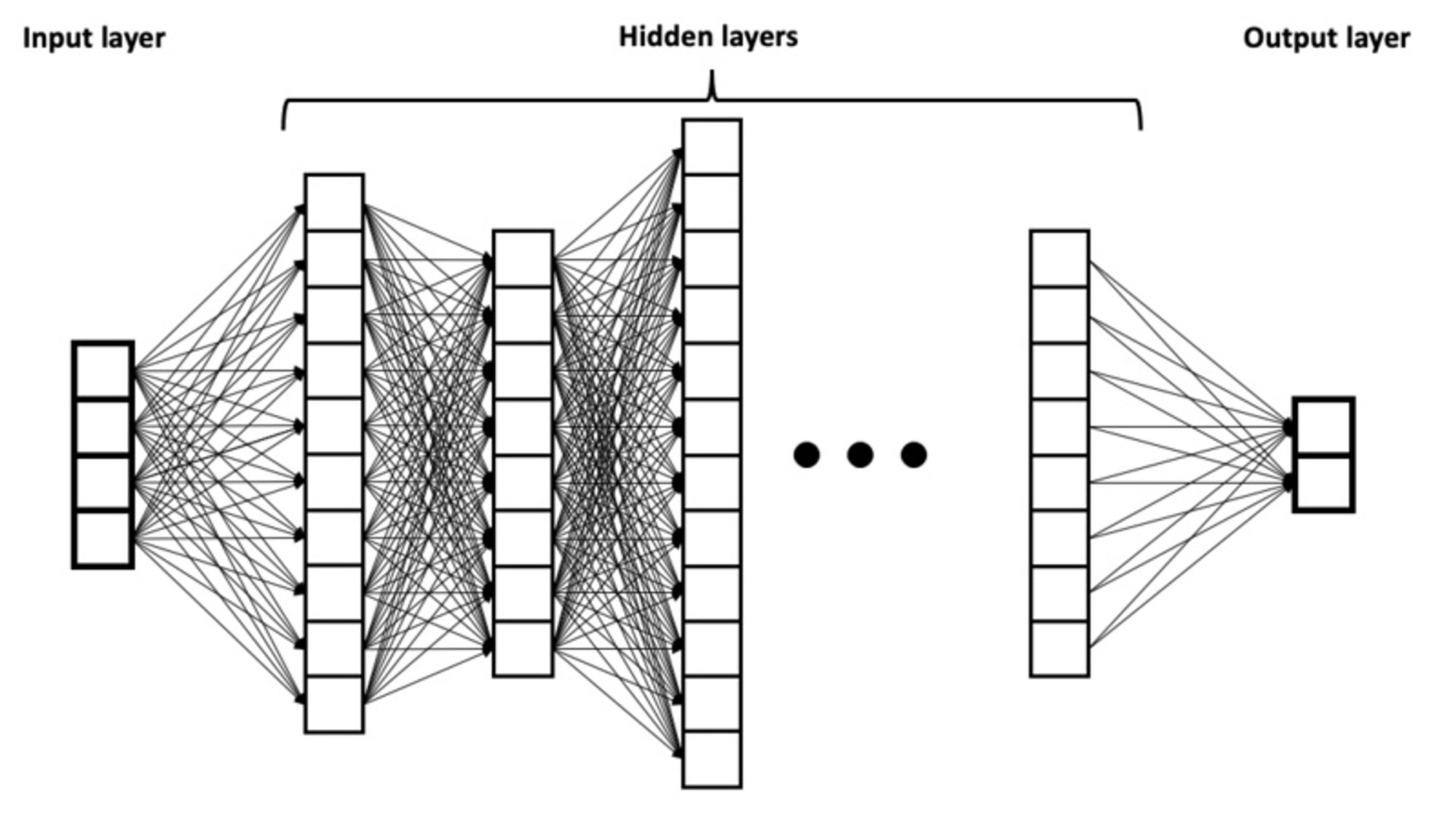
In our example, turning an input image into an output answer (cat or dog) depends on the strength of the connections between neurons, much like in the brain. These connection strengths, also known as weights, dictate how much or how little one neuron listens to another. Training a network, in this case, using images that have been labeled by humans as those of cats or dogs, involves finding values for the weights for all neurons in the network, such that the network classifies the images in the training dataset with minimal error. This process is called deep learning.
In 2012, a deep neural network named AlexNet outperformed all other computer algorithms at an annual image recognition contest. Around the same time, researchers started using deep neural networks to build computational models of the primate visual system. Crucially, AlexNet and these computational models were all feedforward networks: the information flowed one way, from the input to the output. The models of the visual system were good first-order approximations. But they could not account for illusions.
Thatâs because feedforward networks are missing an obvious, crucial ingredient: feedback connections.
AI goes inside-out
One of the first substantial efforts to endow an AI with feedback connections came in 2016, when Bill Lotter, a doctoral student at Harvard, working with his thesis advisors, designed a deep neural network that he called PredNet, short for prediction network. PredNet has both feedforward and feedback connections. Lotterâs intention was to implement the Rao and Ballard model of predictive coding, using the sophistication of neural networks. He designed the network with four layers, with each layer predicting the activity, or the outputs of the artificial neurons, of the layer below. Predictions flowed from the highest layer to the lowest, and errors went the other way. To train the network, Lotter used videos recorded in Karlsruhe, Germany, using a car-mounted camera. The networkâs task: given a certain set of frames from a video, predict the next one in the sequence. PredNet learned to look ahead, one frame at a time.
If PredNet was approximating the kind of predictive coding that happens in our brains, then one obvious question was this: would PredNet be prone to optical illusions too? Lotter put PredNet through the paces.
One of the most intriguing illusions Lotter showed PredNet is the Kanizsa figure. Here is an example:
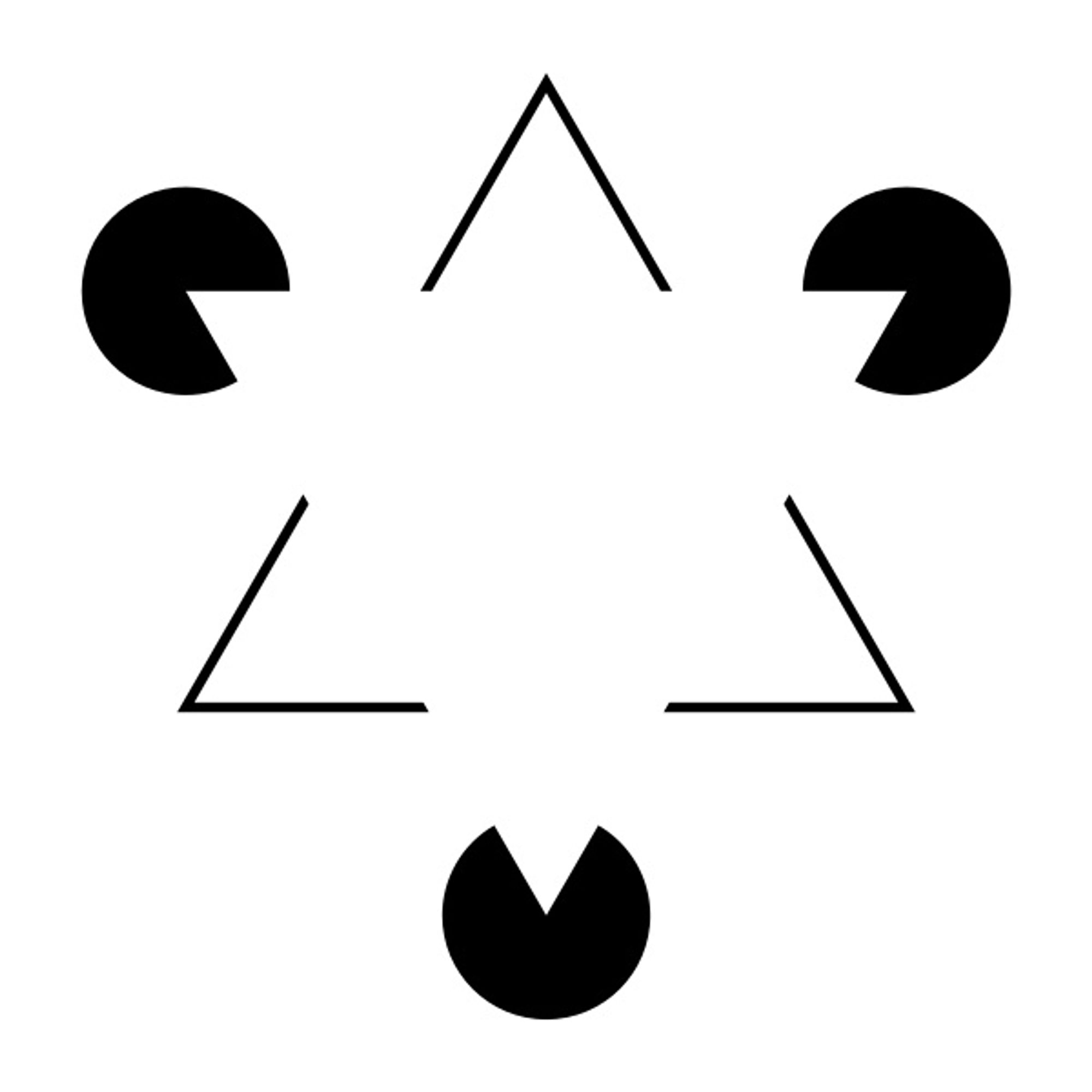
The Pac-Man-like figures are positioned at the ends of an imaginary triangle, such that their open mouths form the triangleâs vertices. What do you see? Itâs a safe bet that you are seeing a white triangle, clearly delineated. Except, thereâs no white triangle there. Itâs been cooked up by our brains. And by cooked up, I mean that there are actual neurons in our brains that are firing in response to the illusory lines; normally, they do so for real lines.
The proof of this comes from monkey brains. In 2001, researchers at Carnegie Mellon University in Pittsburg showed such images to two rhesus monkeys, while recording the activity of neurons from two lower layers of their visual cortex, using surgically implanted electrodes. The tasks for the monkeys were designed to make them pay attention to the illusory figure, bound by illusory contours. The researchers found some neurons responding to the illusory contours. Crucially, neurons in the higher of the two layers responded tens of milliseconds before those in the layer below. This was suggestive of some top-down feedback from the higher to the lower layer (the lower layer should have responded earlier if the processing was simply feedforward). The neurons in the monkeysâ brains were responding to lines, even though there were no actual lines in the visual input.
Inspired by the work, Lotter showed his AI some Kanizsa figures. He specifically looked at the outputs of artificial neurons that usually responded to actual lines in an image. Those neurons also responded to the illusory lines in the Kanizsa figure. Let that sink in: an AI, which has been built to mimic the predictive coding thatâs ostensibly implemented by our brains, is seeing things that donât exist. Wild.
Illusory motion in AI
It was time to ask harder questions of an AI. Could it experience something akin to the moving window sills that I perceived on mushrooms, but without having to feed the AI psychedelics? Even as Lotter was working on PredNet for his PhD thesis, Eiji Watanabe, a computational neuroscientist at the National Institute for Basic Biology in Okazaki, came upon PredNet (Lotter had made the software public by then). Watanabe was impressed. His team wanted to ask other questions of PredNet: would it be fooled by optical illusions that create illusory motion in our brains? To find out, Watanabeâs team first rewrote the PredNet software for their purpose, and trained it using a different set of videos, to more closely model human first-person experience. These videos were recorded by eight people who each spent a day at Disney World Resort in Orlando, Florida, using cameras attached to their caps. PredNet was shown 20 frames of a video and asked to predict the 21st frame. By doing this repeatedly, PredNetâs weights settled into a state that enabled it to make fairly accurate predictions about the next few frames of a video that it had never seen before.
To see if it could predict motion, Watanabe and his team showed their version of PredNet 20 frames of a propeller rotating either clockwise or anticlockwise (three frames are shown below).
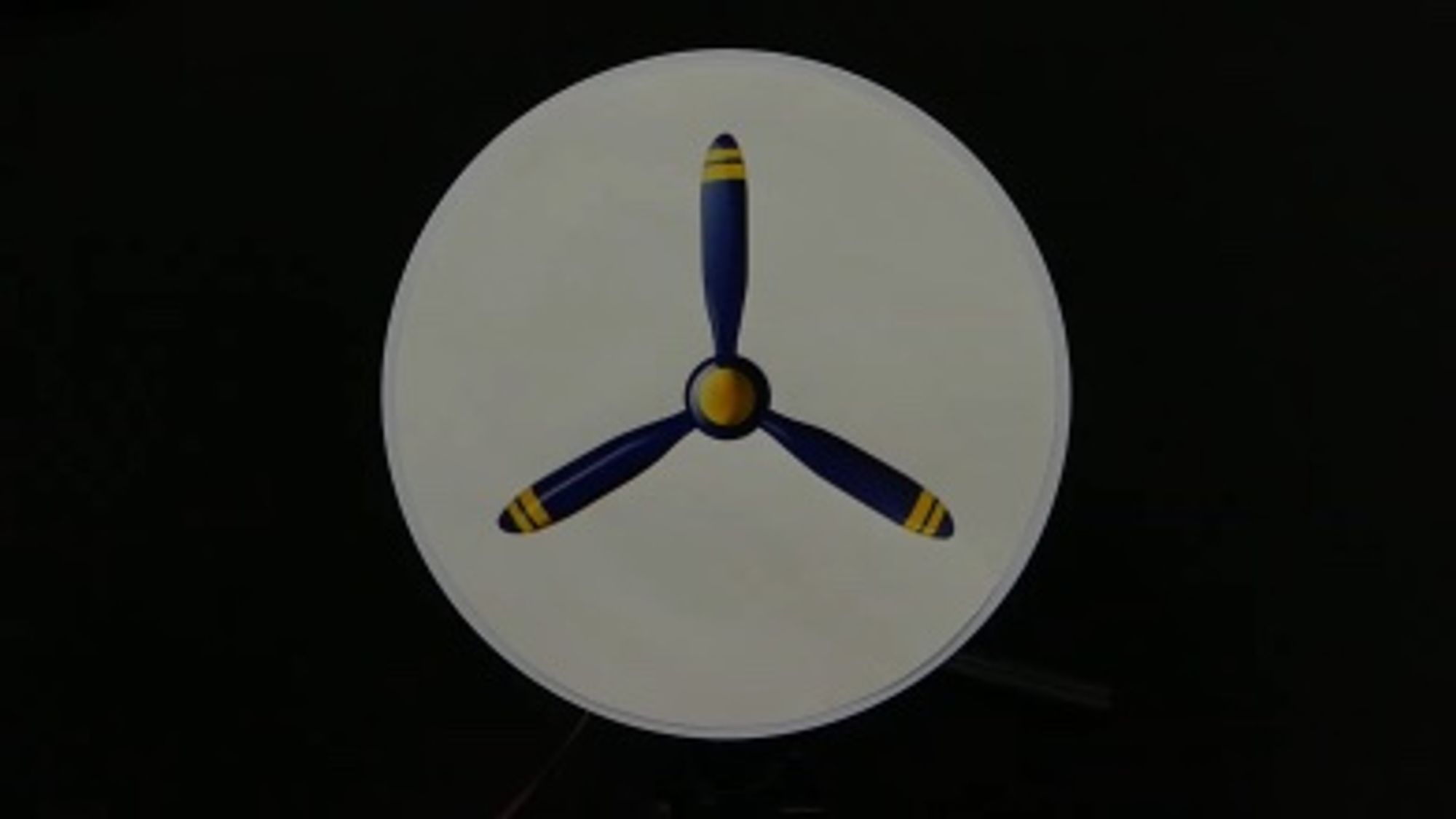
Rotating propellers.
Image: Eiji Watanabe.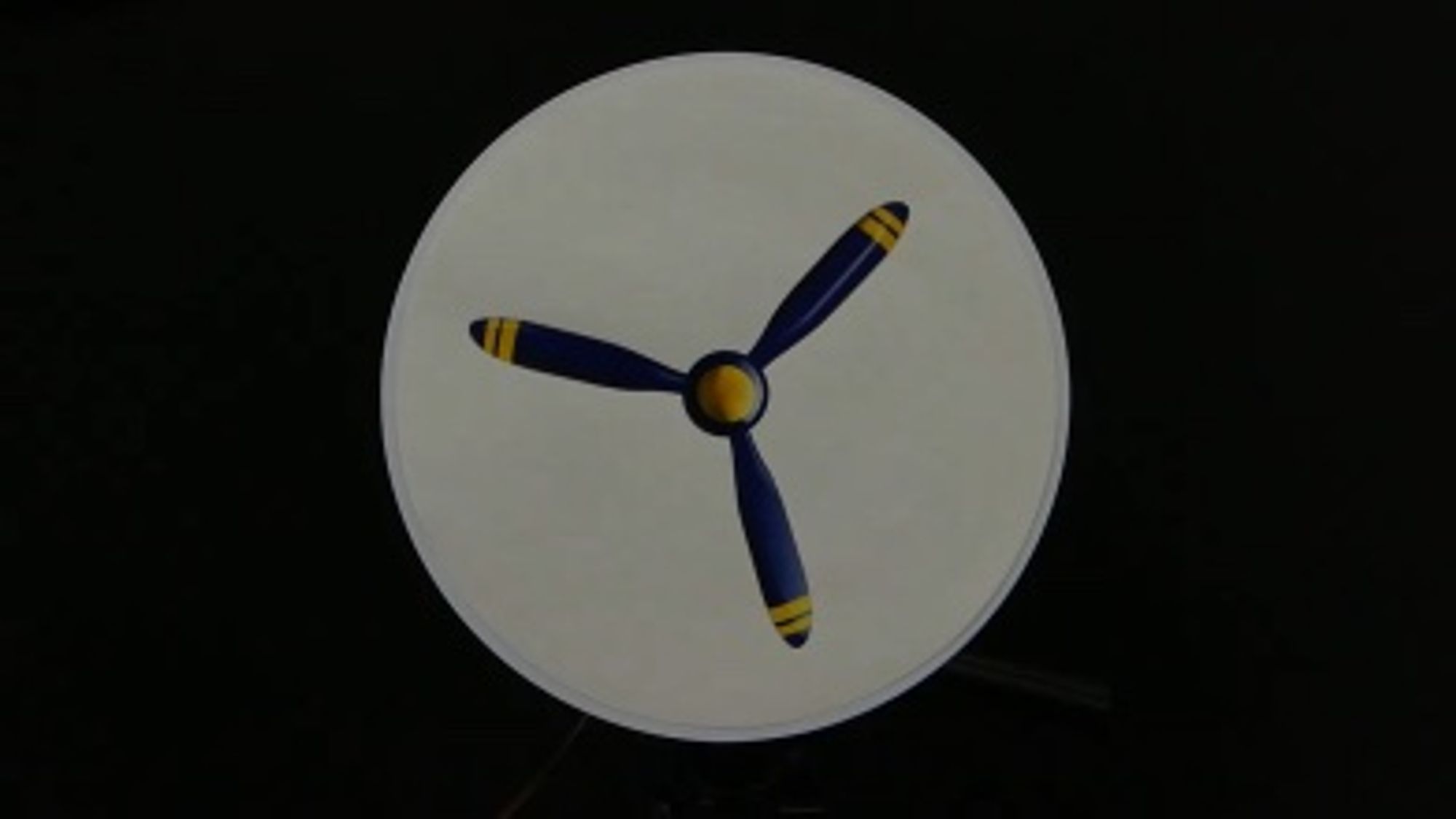
Rotating propellers.
Image: Eiji Watanabe.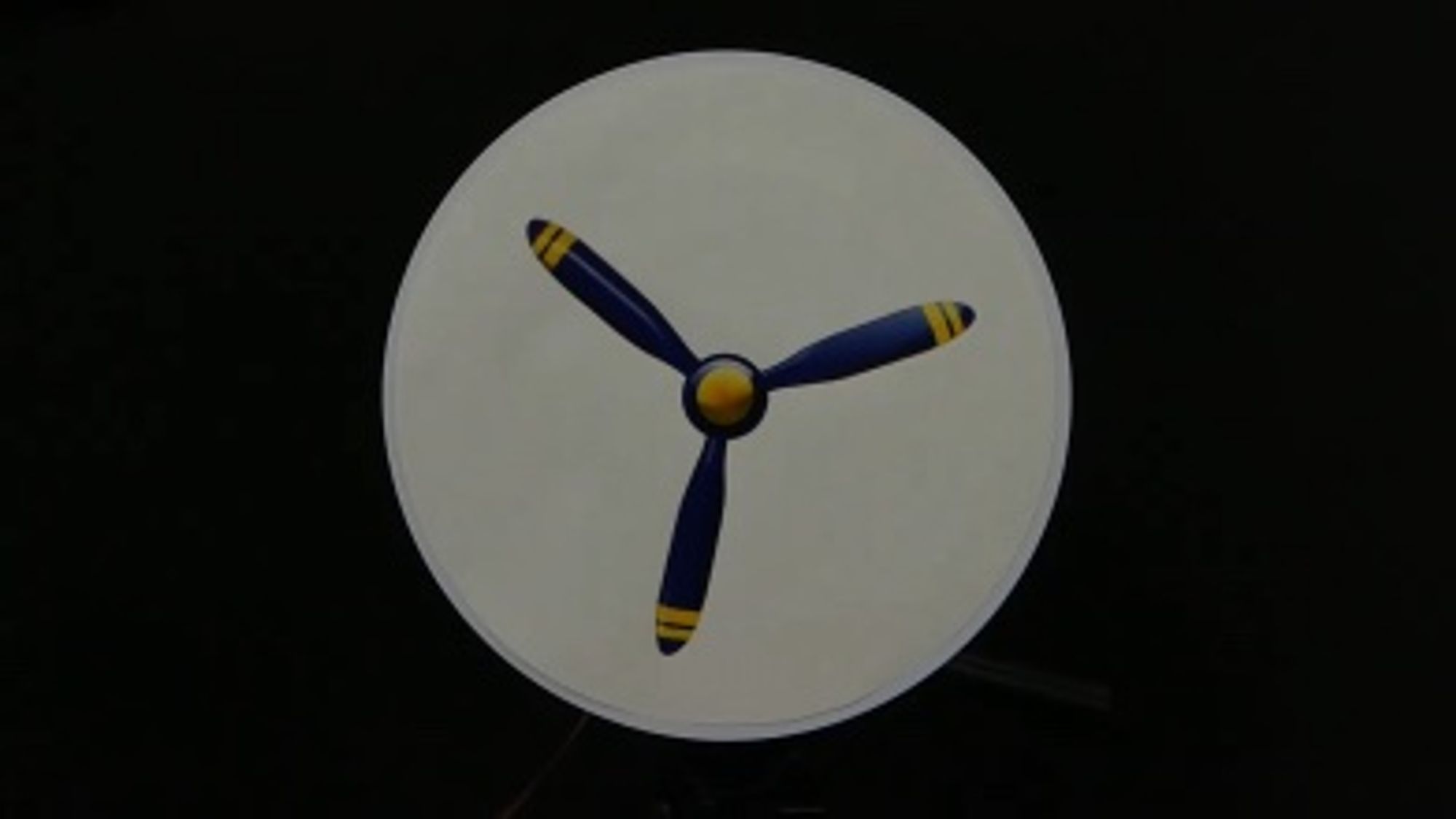
Rotating propellers.
Image: Eiji Watanabe.PredNet got it right: it predicted frames showed the propeller moving in the correct direction.
Next came the acid test: The Rotating Snake Illusion, an extraordinary example of seeing things that donât exist. The illusion was created and published in 2003 by Akiyoshi Kitaoka, professor of psychology at Ritsumeikan University in Osaka, Japan. Hereâs the illusion:
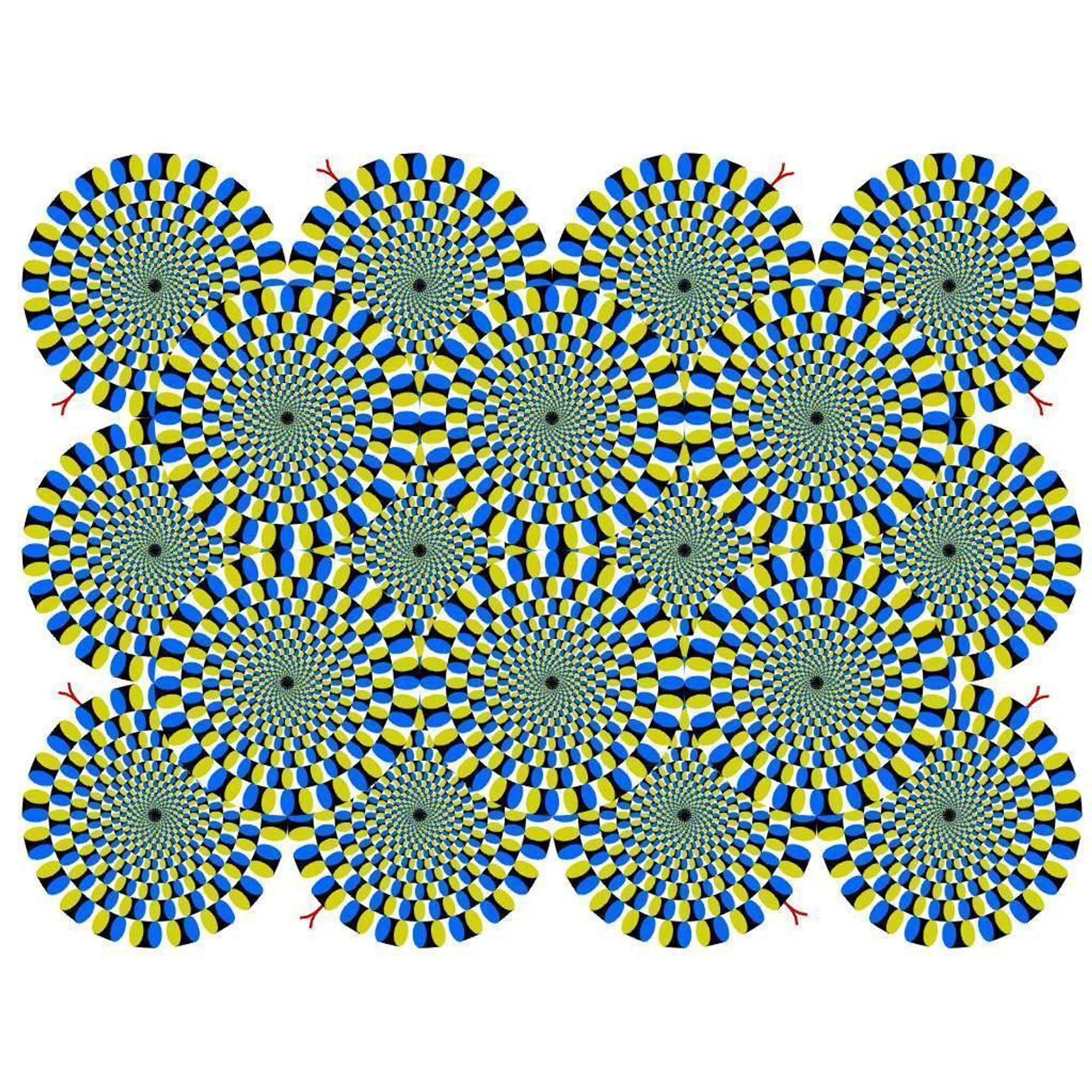
Gaze at the image without focusing on any one region in particular, and youâll very likely see some disks rotating clockwise, others counterclockwise. You cannot stop them from doing so, unless you fixate on any one disk. If you fixate, they all slow down and eventually stop moving. There is nothing in the image thatâs actually rotating, of course. The motion is illusory; something conjured up by our brains, as a best guess of the external causes of visual sensations (neuroscientists think it has something to do with the precise gradation of colors, though an exact understanding of the illusion eludes them). Even cats seem susceptible. They paw at color printouts of the illusion. Given their propensity to pursue movement, itâs likely they too perceive the illusory motion.
Would a predictive-coding AI fall prey to illusory motion? Watanabeâs team showed PredNet a sequence of 20 static images of the Rotating Snake Illusion. Twenty identical, static images. Nothing in those frames was moving. And yet, PredNetâs prediction for the subsequent images in the sequence showed movement. Internally, PredNet âperceivedââif that word can be used for an AIâthe disks to be rotating. âThe network was able to predict movement or motion in the same way as human perception,â Watanabe told me.
Itâs clear that computational models of predictive coding are replicating some of the odd behaviors of our visual system. Rufin VanRullen of the Centre de Recherche Cerveau & Cognition in Toulouse, France, cautions against over-interpreting the results. âThe fact that you can replicate something in an artificial system [doesnât] prove that this is happening in the brain,â he told me. And yet, itâs corroborative evidence. âWhat would have been problematic is if you implemented this in an artificial system and you didnât get the expected behavior.â That would most certainly have been a knock against predictive coding in the brain.
The self as prediction
Given such computational support for predictive processing, we can try and tackle the bigger question we raised earlier. If, as Hume exhorted, we are a bundle of perceptions, and perceptions themselves are essentially hypotheses, is each one of us simply a hypothesis? The rather emphatic answer from some neuroscientists who take predictive coding seriously is: yes. Our sense of selfâthe feeling we have of being a body situated in 3D space with a first-person perspective on the worldâis a coming together of hypotheses that our brains conjure up to make sense of all the inputs, from within the body and without. And these hypotheses are based on prior beliefs and conditioning. The more certain the brain is of its priors, the sharper and more stable the perception.
When I took mushrooms, my perception of myself blurred. I felt less distinct. My brainâs confidence in its hypothesesâthe very hypotheses that constituted meâhad dissipated. Oddly, it was accompanied by a curious pleasantness. But I donât want to romanticize the blurring of the boundaries of the self. I knowâfrom having talked to many people who have suffered from schizophrenia, depersonalization disorder, and other neuropsychological conditionsâthat the breakdown of such boundaries can be traumatic. Anecdotes about similarly scary trips on psychedelics abound. And yet, I cannot help but wonder whether I am a prediction, a perception conditioned by prior beliefs and experiences. Referring to the pleasing blurriness, I asked my friend, who was chaperoning me during my mushroom trip: âWhy donât I feel like this always?â Why indeed. Somewhere in the story of how the brain creates perceptionsâa story created by connecting seemingly disparate dots between Helmholtz, computational neuroscientists, optical illusions, and modern artificial intelligenceâI feel there are answers to these questions about the nature of the self and why we donât always feel light and fuzzy the way I did that day. âŚ
Subscribe to Broadcast