Algorithmic Apparitions
When I think of my own family photo albums, I imagine a thick, tactile cover, yellowing end papers, and page after page of rectangular photos, sorted and slotted into their laminated covers. But when artist Aarati Akkapeddi shows me their family photos, they pull up a Finder window on their desktop and click through dozens of tiny blue folders. Meticulously labeled by person and occasion, Akkapeddi’s digital organizational system is one of preservation and remembrance, but it’s also generative, in service of something new.
For several years, Akkapeddi has been collecting hundreds of photos from family members, scanning them, and sorting them—a process combining both automation and manual labor—then training a Generative Adversarial Network (GAN) on them to produce a composite of each person’s digital likeness. Inputting several decades’ worth of photos of their mother, for instance, results in a morphing portrait that bears a striking resemblance to the subject, while still seeming to search for her features. Fuzzy and unfixed, a spectre more familiar than accurate, the composite image looks uncannily close to how memory feels.
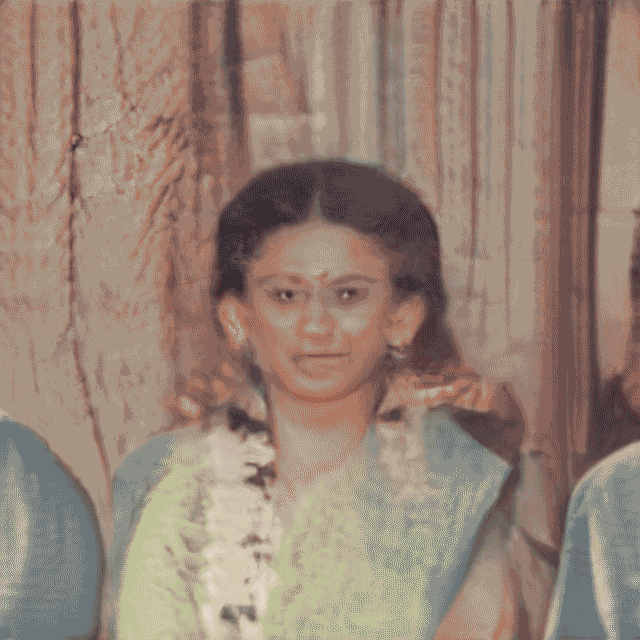
The images above are the beginning of a new project that’s also an extension of an older one. For last year’s Ancestral Apparitions, Akkapeddi mixed family photos with images from The Studies in Tamil Studio Archives and Society (S.T.A.R.S), a research collective archiving South Asian studio photography between the 1880s and the 1980s. They used the machine-generated results to create negatives that they then developed on expired photo paper, returning them to their original medium. The resulting prints are beautiful and haunting, almost Thoughtographic in aura—and they’re some of my favorite visual representations of intergenerational memory, which is a thread that runs throughout Akkapeddi’s work.
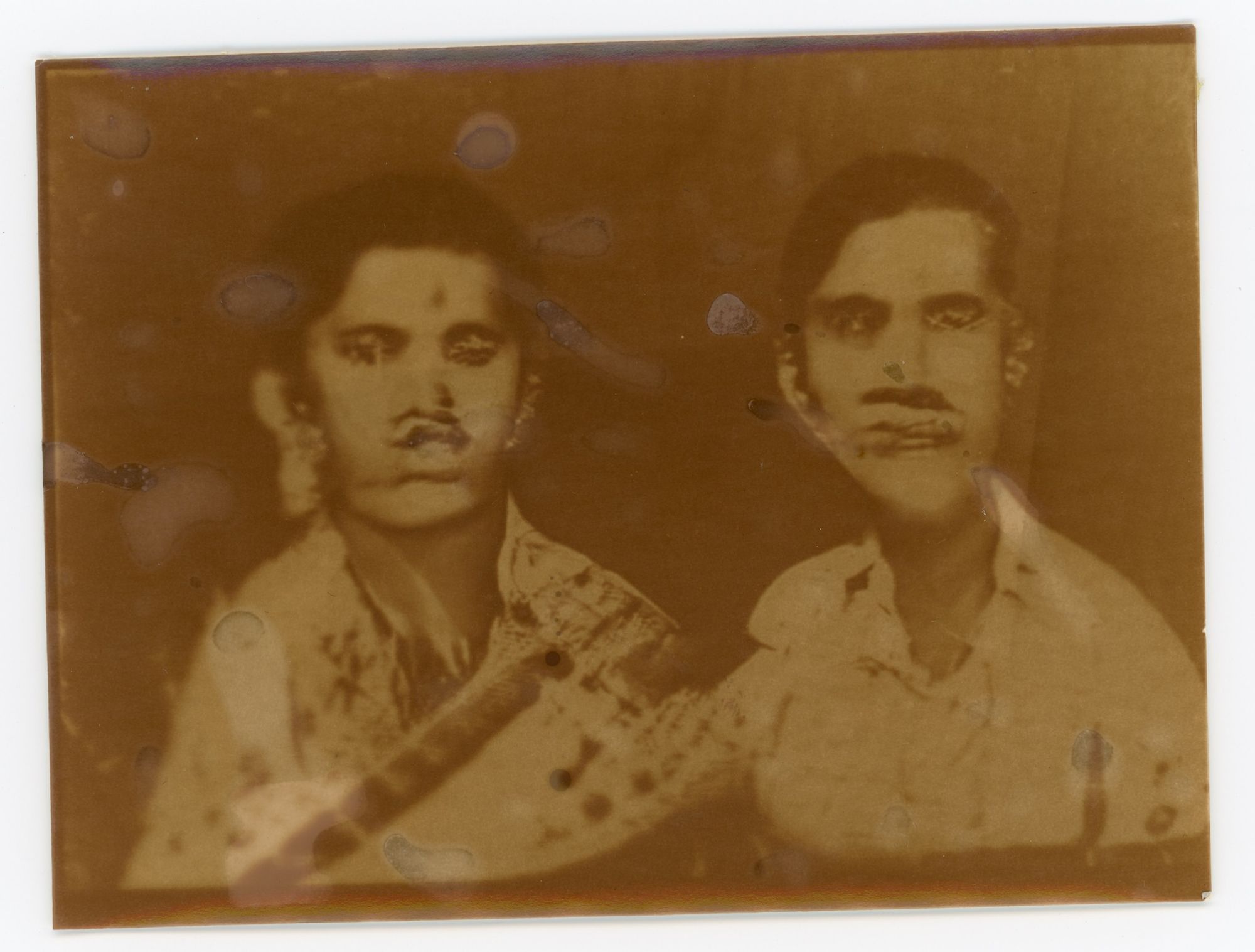
To hear Akkapeddi describe it, machine learning is a fitting medium for the subject of memory, which is “productive in the sense that you're taking scraps of information, whether firsthand experiences or stories you hear, and piecing together a narrative that will ultimately always be different from reality.” Because they're using a relatively small dataset, the aesthetic generated by these algorithms is ghostly and vague, mirroring the malleable quality of our memories. There’s a fictive element to the things we remember, the stories that we create to explain ourselves to ourselves, and that comes through in the generated photographs that are not, in fact, photographs. “At the end of the day, it's not actually an image of [my mother],” Akkapeddi says. “It's just a bunch of pixels that are arranged in a certain way.”
These pixels were arranged by a GAN, which is essentially a machine learning system that’s used to generate synthetic imagery. Many people know GANs via their association with the phrase “This ___ Does Not Exist,” thanks to a collection of websites showing how a GAN can create photorealistic versions of just about anything. It’s a technique that attracts both artists excited by its potential as a creative medium, as well as more sinister actors interested in generating deepfakes and spreading misinformation.
GAN systems are comprised of two networks: one known as the “generator,” and the other the “discriminator,” which are pitted against each other (giving us the “adversarial” in generative adversarial network). The “generator” tries to create images that appear as close as possible to the data it’s trained on, while the “discriminator” compares the generated images to the real images, sending the “generator” back to the drawing board until it can no longer distinguish between the real and unreal. Throughout the process, the model is discovering and learning the regularities or patterns in the input data so that it can better generate the outputs.
In their work with GANs, Akkapeddi pays close attention to these patterns as they emerge, because they allow them to see “compositional throughlines” in the data that they might have otherwise missed. They could start to notice, for example, that in the studio portraits collected by S.T.A.R.S, people are commonly posed a certain way. They can then take it further in that direction by removing images that do not fit this pattern from the dataset, ultimately influencing the output images so that they will show that same pose. “In that way, it's also about finding what’s shared,” Akkapeddi says, “both culturally and aesthetically.”
This practice of bringing the human hand into the process to help determine how the generated images come out, rather than leaving it entirely up to artificial intelligence, is what Akkapeddi refers to as “foraging and forging.” “Foraging” refers to the act of hyper-curating their dataset: only family photos that align with a certain pattern, or only photos of their family, or only photos of their mom. Working in this way makes the data itself a point of creative control, which can then shape the aesthetics and content of the outcome (“forging”). While the GAN adds serendipity and randomness to Akkapeddi's artistic process, the human process of selecting images, scanning them, watching for patterns, and weeding out outliers acts as a kind of creative constraint. Similar to the poetic constraints used by the French literary group Oulipo or the automatic writing practiced by Surrealists, the foraged dataset is a technique that binds the project to certain conditions, revealing new patterns and perspectives in the process.
It’s also a process that’s highly subjective, which Akkapeddi says has given them a better understanding of machine bias, or the biases of harm ingrained within machine learning—which is also to say, the biases of the people collecting the data AIs are trained on. As the artist Allison Parrish has written, “Although data seems inert, it is never neutral. Data directly results from the ideology of those that gather it, and it records the physical and cultural contexts of its collection.” In other words, we influence the systems we create, and this is something Akkapeddi embraces, even takes advantage of. They're interested in exploring how machine bias reveals our own biases and tendencies, and in turn, how machine learning could be used as a catalyst for self-reflection.
In March, I attended a talk that Akkapeddi gave to design students at Rutgers University, where they spoke about Ancestral Apparitions alongside works like their stunning Kolam series, in which they use facial recognition data to create looping patterns inspired by the Indian art form of kolam, then emboss them onto family photos. Akkapeddi has long been interested in developing methodologies for translating data from one form to another, but using machine learning is relatively new for them. When they got to their latest work in the talk, I was intrigued to hear them liken their practice of curating and intentionally editing datasets to “leveraging machine bias.”
Since AI systems learn to make decisions on training data, biases arise from those datasets: it can result from flawed sampling data that over represents a certain group, or be shaped by human decisions and broader historical or social inequities. As our daily lives are increasingly mediated by artificial intelligence, bias shows up everywhere from predictive policing to job recruiting and photo tagging. Left unchecked, it has the potential to do a lot of harm, particularly toward people of color and marginalized identities, which is something many visual artists, designers, and creative technologists have helped illuminate.
Joy Buolamwini’s work, and in particular her project Gender Shades and spoken word poem AI, Ain’t I A Woman, reveals how facial recognition technology misgenders Black women. Sasha Costanza-Chock’s work and writings have made visible the biases against trans people that are ingrained in airport security technology. And Simone Browne’s book Dark Matters, which traces how surveillance technologies and practices are informed by the history of racism, has inspired artists such as American Artist and Neta Bomani (see: Bomani’s video-zine Dark matter objects: Technologies of capture and things that can't be held).
While drawing influence from some of these artists, Akkapeddi’s work approaches the subject of machine bias less directly, instead exploring it through the filmy lens of familial memory. I wondered how Akkapeddi was using something as insidious as bias as a means to produce such familial, intimate, and beautiful works.
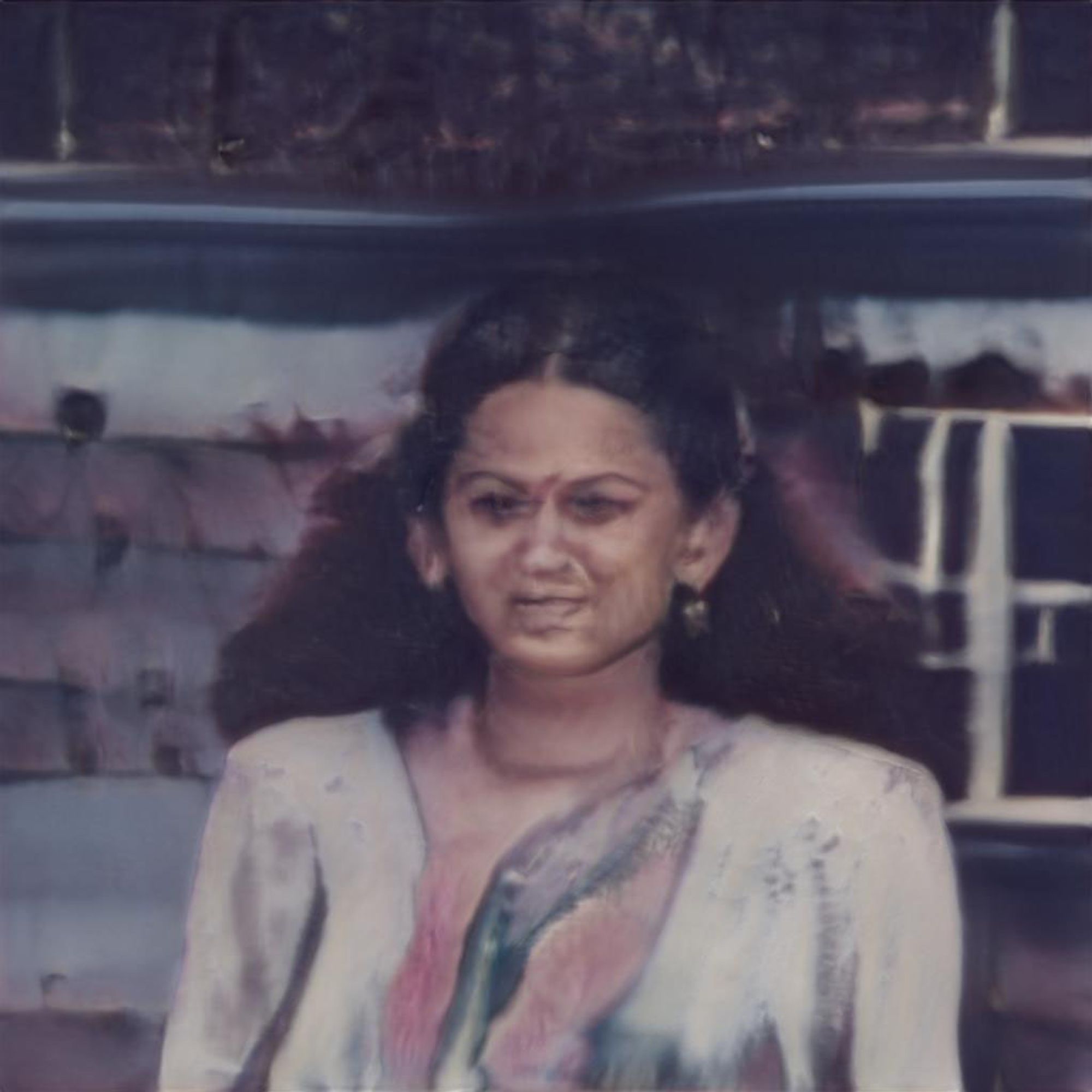
I knew if I walked in your footsteps, it would become a ritual, 2021. Machine generated portrait of Akkapeddi’s mother, produced as part of a residency with Ada X in Montreal.
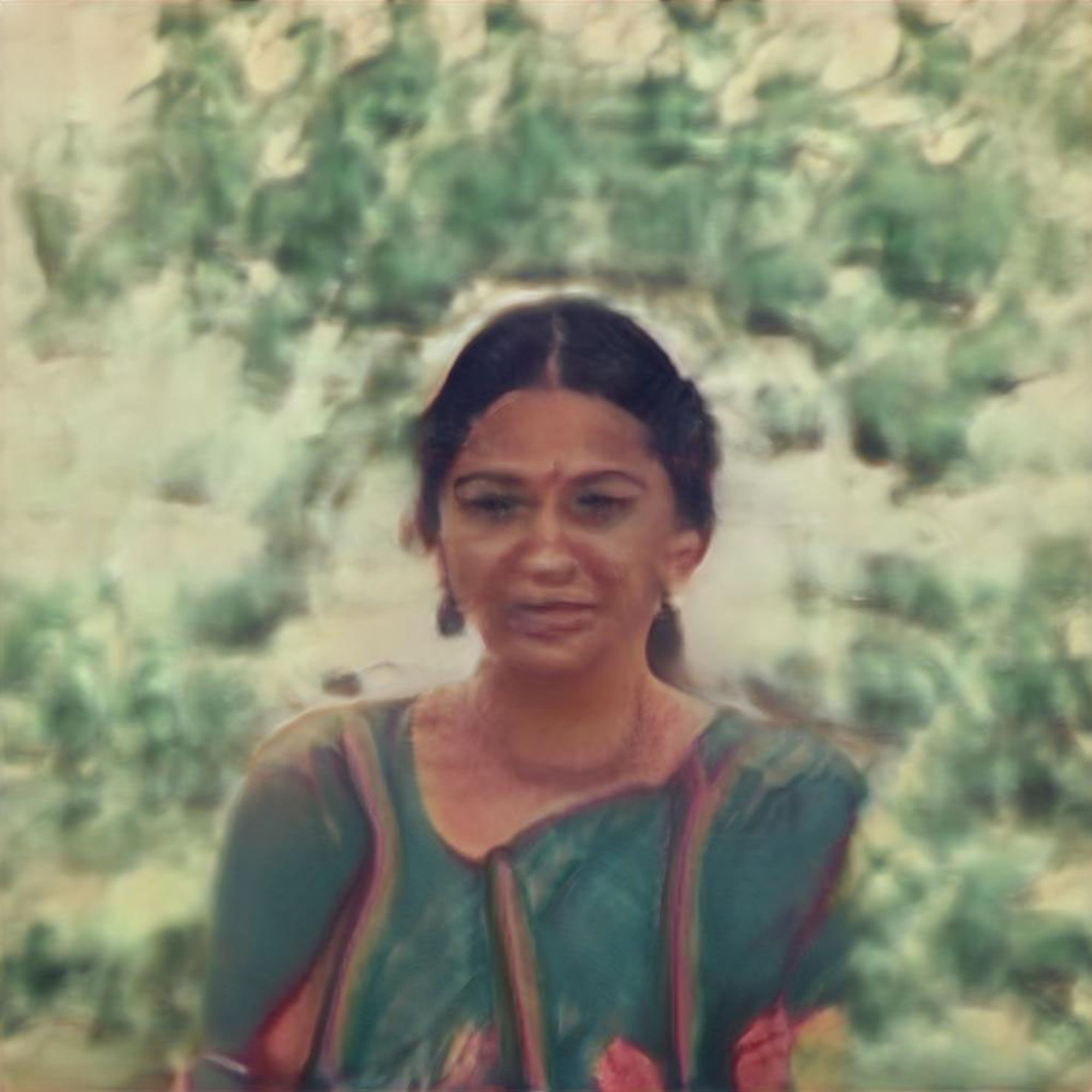
I knew if I walked in your footsteps, it would become a ritual, 2021. Machine generated portrait of Akkapeddi’s mother, produced as part of a residency with Ada X in Montreal.
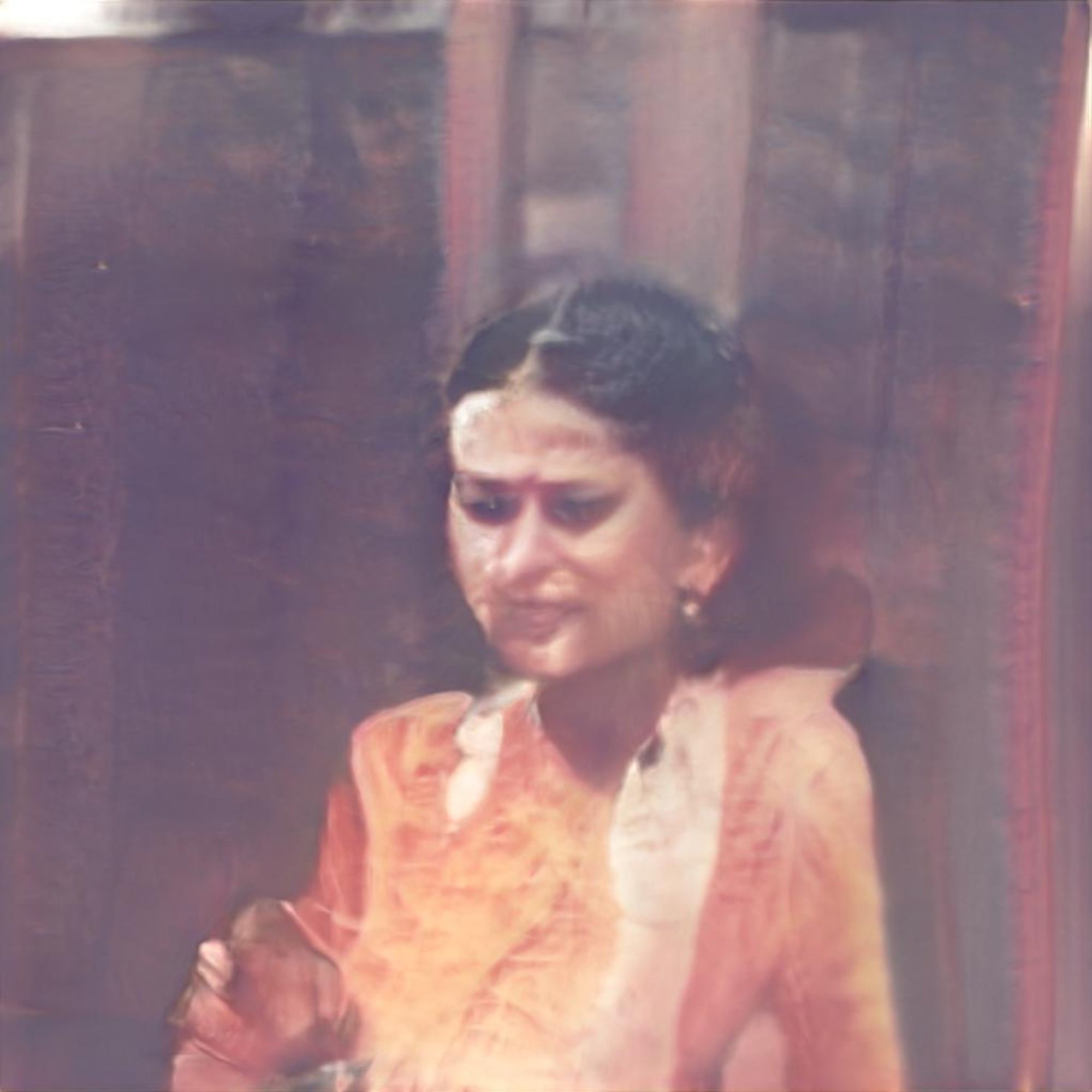
I knew if I walked in your footsteps, it would become a ritual, 2021. Machine generated portrait of Akkapeddi’s mother, produced as part of a residency with Ada X in Montreal.
“Sometimes I struggle with that tension of the coldness associated with the technologies that I use in my work and my intention which is much more personal,” they tell me when we meet over video call. “I think for me, I'm drawn to machine learning because it feels like a way to reflect.” They tell me about the commonalities that the GAN they've trained on their family photos has revealed to them about the material they know so well. After using a facial recognition algorithm to align the photos by face, Akkapeddi shows the images to the GAN, which finds patterns and generates its own approximations of the images. As they watch the model produce images of their mom dressed in yellow, they realized it was because of the photos of their mom’s wedding and the yellow sari she wore. When Akkapeddi segmented the larger dataset by event, they saw that weddings were by far the biggest source of photos (they have over a hundred of their parents’ wedding alone). “I realized that’s for a reason: I’ve experienced it firsthand, the pressures, the type of value that's placed on marriage for women in my family,” they say.
After noticing such trends, Akkapeddi decided to start interviewing members of their family about the photos, and incorporating their conversations into the project as a way to supplement the narratives arising from their own sorting and segmenting. Talking with their family helps them recognize inconsistencies in their own memory, like with photographs of their grandfather they could have sworn were taken in Oklahoma, where he first immigrated, but which were actually taken in his hometown of Vijayawada in the Indian state of Andhra Pradesh. These conversations also reveal their own discrepancies, like when Akkapeddi's grandmother’s account of the circumstances surrounding a certain photo will differ from their mom’s account, a by-product of the messiness of intergenerational memory.
The addition of the interviews to the project reminds me of the work of writers like Nathalie Léger and Saidya Hartman, who use archival work as a starting point from which to develop their own narratives. In both cases, these writers are working with fragments, the scant documentation and material remnants of lives historians and archivists didn’t deem worthy of full preservation. In Hartman’s Wayward Lives, Beautiful Experiments, for example, she tells the “intimate histories of riotous Black girls, troublesome women, and queer radicals” in the early 20th century by connecting letters, photographs, and sociological data with her own fiction and narrative non-fiction. This methodology, which she terms “critical fabulation,” has the dual effect of bringing to life stories that were lost, while also critiquing the private and institutional values that control what is collected and preserved.
Akkapeddi’s work can also be seen as a critique, less of the archive as a non-neutral space as it is of the dataset an algorithm is trained on. They critique the human subjectivity inherent in data by leaning all the way into it, and seeing what the outcomes reveal about ourselves. “With art, I'm interested in the idea that machine bias reveals our own bias, and I think that can actually be quite a useful thing,” they say. While acknowledging that they don't know how applicable this thinking can be to practical uses of machine learning—at least under capitalism, where “the chief concern for those applications is for efficiency over ethics”—Akkapeddi believes accepting that data is reflective of our own biases and subjectivity can be a powerful tool for revealing the holes in histories, both individual and collective, that we may not otherwise question. “[Machine learning] doesn't just breathe a new life into the archive, but rather births something new,” they say. “I believe our memory works in a similar way. It's not documentation, it’s loaded with subjectivity and emotion.”
Subscribe to Broadcast